Driving success for pioneering biotech companies with AI and big data
- autumnlee31
- Apr 2
- 5 min read
AI is changing the future of medicine - read on to discover how one of our companies has developed a ‘research engine’ to drive success in drug discovery efforts.
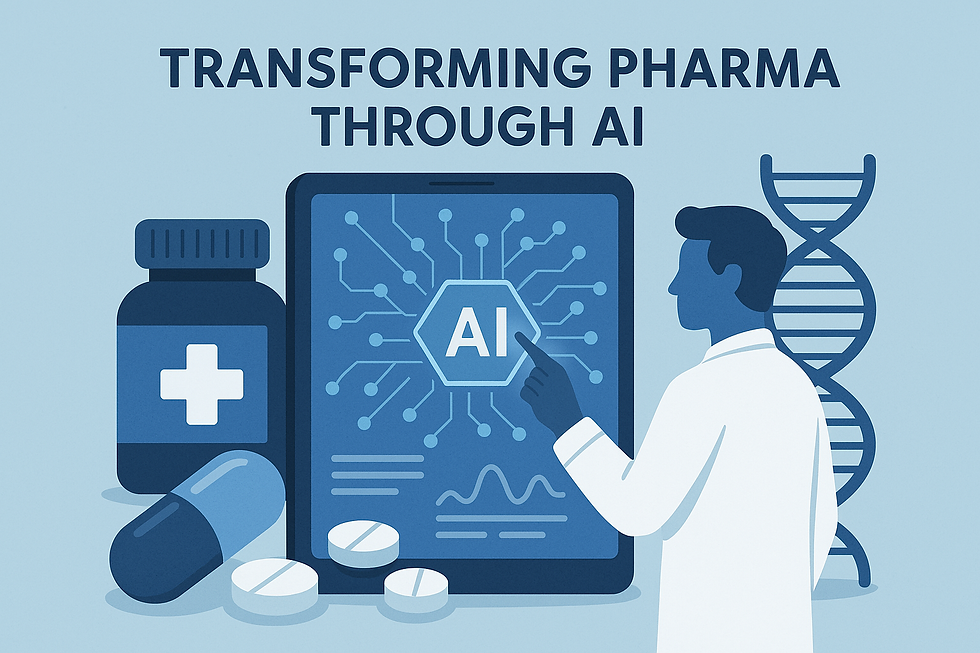
A game changer in drug discovery
A key element of General Inception's strategy has been its acquisition of Enable Medicine and its powerful generative Al platform. Reddy highlights the significance of the 'Enable Atlas,' comprehensive collection of clinical and biological data: "Enable Medicine has built one of the most extensive clinical and biological datasets in the world, termed the Enable Atlas." This vast resource is the foundation for Enable's Al platform, which Reddy describes as an "insight engine".
The platform sifts through patient care journey data, identifying complex interactions between cells, proteins and genes that influence disease progression and responses to therapy. "Using Enable's unique Al platform, we leverage the Atlas to comb through vast amounts of data from a patient's care journey," says Reddy. By employing Al models capable of efficiently testing thousands of hypotheses, the platform generates predictions about patient responses to specific drugs and uncovers novel drug targets in specific patient subgroups. "Using Al models that efficiently test thousands of hypotheses, we generate predictions about which patients will be most likely to respond to specific drugs and discover novel drug targets in high-need patient subsets."
These predictions are not merely theoretical, they are rigorously validated through ongoing clinical trials. Reddy highlights studies such as the combination immunotherapy trials led by cancer immunotherapy pioneer and Nobel Laureate Jim Allison, alongside Dr. Pam Sharma at the MD Anderson Cancer Center in Texas. The insights derived from the Enable Atlas and Al platform have a ripple effect throughout General Inception's portfolio of biotech companies. "Enable serves as an insight engine, and this engine gives all of the therapeutic programmes across our current portfolio of 13 biotech companies increased odds of success throughout their development pipelines - from discovery to clinical positioning," he explains. This approach, Reddy emphasises, improves target selection and patient matching, significantly increasing the probability of success in clinical trials. "This approach not only helps us select targets with real therapeutic potential but also match them with appropriate patient populations to greatly increase the odds of success in clinical trials."
Navigating the challenges of Al in biological data analysis
While the potential of Al in drug development is immense, Reddy acknowledges the challenges involved. He outlines General Inception's multi-pronged approach to overcoming these obstacles, focusing on data quality, expert involvement, model interpretability and validation. "Leveraging Al for drug development presents challenges, but we've adopted strategies to navigate them. These include building high-quality data, engaging expert scientists in an iterative machine learning process, avoiding black box models, and validating hypotheses computationally and experimentally."
High-quality data is paramount. "Our models rely on carefully curated datasets that connect patients and their outcomes to underlying biological processes. This requires substantial infrastructure investment to support data collection and ensure quality control." Reddy stresses the critical role of expert scientists in refining the Al models. "Al models have inherent flaws and none are completely accurate. But we establish benchmarks to evaluate model performance, engaging highly experienced specialists including immunologists and oncologists to help refine prediction loops. Their input helps correct errors, greatly improving our models over time." He emphasises that achieving the right balance between efficiency and accuracy demands additional investment in data annotation and review tools. In addition, he highlights the critical role of peer review through academic publications, citing the example of benchmarking methods for labelling tissue niches.
General Inception also prioritises interpretable models over 'black box' approaches. In Al and machine learning, a black box model might generate accurate predictions or results, but it is difficult to understand why or how the model arrived at those conclusions. "While black box models may suffice for straightforward predictions, biological enquiries demand a mechanistic understanding. We favour interpretable models, like graph neural networks, which elucidate the features driving predictions, grounded in our understanding of biological components."
Reddy also emphasises that Al is a tool for hypothesis generation, and not a replacement for empirical validation. "We view Al as a tool to generate hypotheses and analyse vast datasets. Our approach enables us to narrow these hypotheses down to testable ideas that can be validated empirically, rather than accepting Al output blindly."
Autoimmune and inflammatory diseases
General Inception's recent acquisition of Enable Medicine is yielding significant results, particularly in autoimmune and inflammatory diseases. "Enable Medicine leverages curated data maps and advanced Al to efficiently answer biological questions. In autoimmune diseases, conserved pathways worsen inflammation. Early-stage biotechs often face the challenge of determining which indications to target with their molecules," informs Reddy, contrasting this with traditional methods, which can cost significant time and money. "Traditionally, answering this might involve a lengthy and expensive process of collecting data from various indications, profiling relevant pathways, and analysing the data to identify suitable indications. However, using Enable's comprehensive disease data maps and rapid query models, Enable can significantly reduce the time needed to identify enriched tissues, indications, or cell types related to a drug target."
Influences on drug development strategy
Reddy shares some concrete examples of how the collaboration has shaped General Inception's drug development strategy. "Noting everything I have described above, we have used Enable's Al platform to help select targets for the arms of bi-specific and tri-specific molecules we are developing which target various immune cells in autoimmune diseases." This has led to the identification of novel targets in patients who don't respond to existing treatments like Dupixent (dupilumab), a prescription medicine used to treat chronic conditions that cause inflammation, such as asthma and sinus problems.
"As a result, we have been able to identify truly novel targets in Dupixent non-responders, select expansion indications, and build data packages that provide evidence for further investment into key programmes based on their biological and clinical potential," Reddy reveals. In oncology, the Enable Atlas is also being used to guide the development of natural killer (NK) cell therapeutics.
The future of Al in drug discovery
Looking ahead, Reddy anticipates continued progress in the integration of Al into drug discovery. While acknowledging the spectrum of opinions on the pace of Al's impact, he believes that sustained investment will lead to improvements in infrastructure, data collection, and ultimately the Al models themselves. "There is a spectrum of views out there around how rapidly Al will make an impact on our lives. In drug development, I believe there is still a lot of work to be done to deploy these models effectively and safely, and the evidence that we are really moving the needle will take time to accrue." He continues: "That said, we will absolutely see continued investment in the space which will lead to better infrastructure, better data collection efforts, and ultimately better models that help us make sense of biology and disease. Those who invest early on in the hard work of collecting that data and building that engineering infrastructure, will be well placed to make a material impact on drug development in the future.”
To uncover novel drug targets, Enable Medicine is focused on collecting multimodal data that connects a cell's state and context with the patient's clinical condition. This data, ideally gathered over time and before and after treatments or disease onset, goes beyond traditional sequencing methods. By linking proteins, genes and pathways at the cellular network level, this detailed and context-rich data is essential for understanding the complex mechanisms behind diseases and identifying new potential therapeutic targets.
Transforming pharma through Al
In conclusion, the pharmaceutical industry is changing, driven by the integration of Al and extensive biological data, marking a new phase in drug discovery. While the potential is immense, the journey is not without its challenges.
General Inception is tackling these challenges related to data quality, model transparency and the need for empirical validation, ensuring AI plays a crucial role in drug development. With ongoing investment and innovation, AI integration in drug discovery is set to deliver therapies that could greatly enhance patient outcomes.
Comments